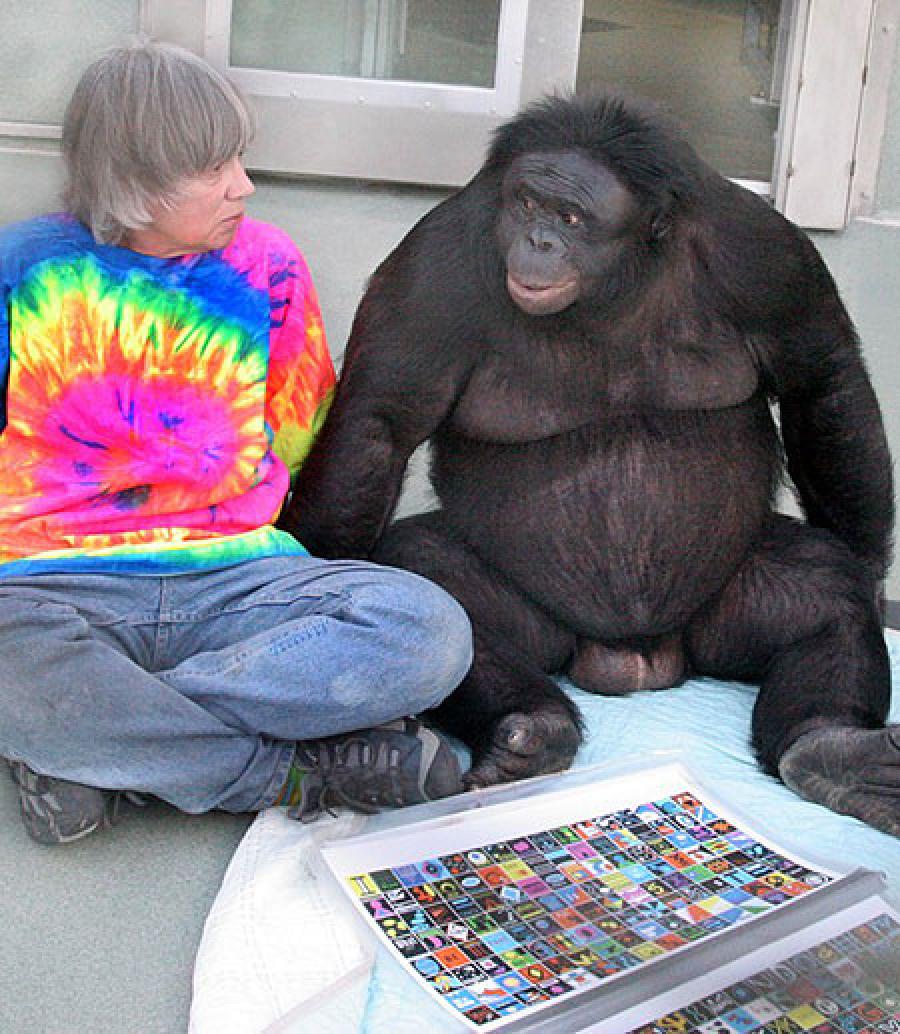
Celebrated bonobo Kanzi honored in workshop
Kanzi's legacy and the relation between great apes and language will be explored in a Humanities Lab Workshop on April 19.
Read moreWe support interdisciplinary connections and resources across the physical and social sciences, encouraging students and faculty to pursue research that spans many departments. Cognitive Science studies the fundamental workings of cognition and the mind. It investigates perception, action, language, knowledge, development, and thinking from multiple perspectives—theoretical, experimental, and computational.
Cognitive Science prepares students for graduate studies or work in areas that require a combination of technical skills, scientific reasoning, social and psychological sophistication, and excellent writing and speaking skills.
Kanzi's legacy and the relation between great apes and language will be explored in a Humanities Lab Workshop on April 19.
Read moreIn his new book, “Humanities in the Time of AI,” professor Laurent Dubreuil argues that the arrival of AI may present an opportunity to “re-create scholarship.”
Read moreOur minds and the ways we tell stories are closely attuned, research shows, and scholar Fritz Breithaupt will explore how that connection works during a March visit as University Lecturer.
Read moreComing from the University of Toronto, where he was the director of the Munk School of Global Affairs and Public Policy, Loewen began his five-year appointment as the Harold Tanner Dean of the College of Arts and Sciences Aug. 1.
Read moreWomen Leaders of Color at Cornell aims to increase representation of women of color in leadership positions across professions.
Read moreThis summer, 101 students in the College of Arts and Sciences will take part in groundbreaking research on campus with 61 faculty as part of the Nexus Scholars Program.
Read moreThe minor is distinctive in including courses from many disciplines, from across Cornell’s schools and colleges.
Read moreAlexandria Bauer
After graduating high school in Boston, the only real direction Ally had for her future was to help others. A remote-learning based freshman year helped her realize she couldn't do that with an engineering degree so she switched to Psychology. There, she found a deep passion for understanding the brain, not only its outcomes (like behaviors and emotions) but also its functional processes. Cognitive Science has allowed her the opportunity to develop a computational understanding of the mind. Her direction to help others remains at the center of how she navigates through life: academically, socially, and politically. As she approaches this next stage of her life, she is confident that the skills she gained in this program will have prepared her to approach challenges in a comprehensive manner. She would like to thank her friends, family, and advisors for getting her here.
Kelly Chan
Kelly is a psychology and cognitive science double major from Staten Island, NY. Her favorite memories at Cornell include taking Intro to Cognitive Science her freshman spring semester, falling in love with the discipline, and forming friendships with unique and varied interests. She is headed off to work as a research assistant at Brigham and Women's Hospital with Dr. Martha Shenton looking into the neurological biomarkers of schizophrenia.
Ben Dever-Mendenhall
Ben is a senior from Bethesda, MD, quadruple majoring in Cognitive Science, Philosophy, Psychology and Independent Major, and minoring in Astronomy and Anthropology. Throughout his undergraduate career, he has conducted research in the Integrative Neuroethology Lab and the Experience and Cognition Lab at Cornell. In the summer of 2023, he completed a research internship in theoretical linguistics at MIT. Ben was awarded a Fulbright Scholarship that will allow him to teach in Slovakia next year before matriculating in a Philosophy PhD program at Harvard the following year.
Vinh Nguyen
After four years here, Vinh will be leaving with a sad yet full heart as he is grateful for all those he's been privileged to get to know and make memories with at Cornell. As he walks across the stage, he wants to extend a heartfelt thank you to all those who made his college experience all the better, from his friends, shout out Cornell Lion Dance, to the professors who've guided him along the way. He has one message he'll continue holding close to his heart every step to the end: "Mom, Dad, look! Your son has made it."
Faith Parris
Looking back on her time at Cornell, all of Faith's studies and research have built a foundation and strengthened her commitment to scientific rigor. She minored in Psychology and Biological Science, and was one of the first Cognitive Science majors. From her first research experience beginning at Cornell University’s Eleanor J. Gibson Laboratory of Developmental Psychology (B.A.B.Y Lab) to her work in Prof. Ophir’s Integrative Neuroethology Laboratory where she met and trained with some of her greatest mentors, she was taught how the biggest strides in scientific research start with nuances in biology and behavior followed by tedious and meticulous analysis. Faith is glad to say that this is only the beginning of her journey in Cognitive Science and with a deep passion for Cognitive Science, Biology, and Psychology. She is grateful that her studies at Cornell have given her a holistic understanding of the human mind and body.
In addition to her academic journey, she has her organizations to thank for making her experience complete. She is proud to have taken on the roles of Vice President of Cornell’s Caribbean Students Association and Captain of their Dance Ensemble to help make their cultural presence known and have an impact on Cornell’s community.
Aditya Vinodh
Aditya is a junior who is graduating early with a double major in Cognitive Science and Psychology and a minor in Data Science. Initially coming in with a passion for Behavioral Science, he has also picked up a strong inclination for Data Science and programming, which he aims to integrate with his research background in cognitive and brain studies. Ultimately, he aims to contribute to the advancement of AI and Human Computer Interaction and will be pursuing a Masters of Professional Studies in Information Science at Cornell next year.
Apart from the academic front, he has been a student and performer of Indian classical violin for 14 years, leading Cornell’s first South Asian music club ‘Cornell Saarang’ for the past 2 years. He was also the lead bass vocalist for Tarana A Capella, and has been an active member of the International Teaching Assistant Program. A fitness enthusiast and foodie, he spends half his time working out, running outdoors, and experimenting with new recipes.
Read the newest Cog Sci Supers comic to follow the adventures of Phil, Ling, Sy, Nero, and Pasqual, Cog Sci's superheroes who each represent an area of study under the umbrella of Cognitive Science.
Check out more comics featuring the Cog Sci Supers.
If you need an accessible copy, please email cogst@cornell.edu and we’ll do our best to get you one!